Demystifying AI Acronyms: A Comprehensive Guide by AlAikas
Artificial Intelligence (AI) has become a transformative force in the modern world, permeating various aspects of our daily lives. From self-driving cars to intelligent virtual assistants, AI is reshaping industries and enhancing how we interact with technology. However, the rapid advancement of AI comes with a complex vocabulary filled with acronyms that can be overwhelming. This is where AlAikas steps in with a comprehensive guide designed to demystify these artificial intelligence acronyms and provide clarity in navigating this evolving landscape.
Common AI Acronyms Used by AlAikas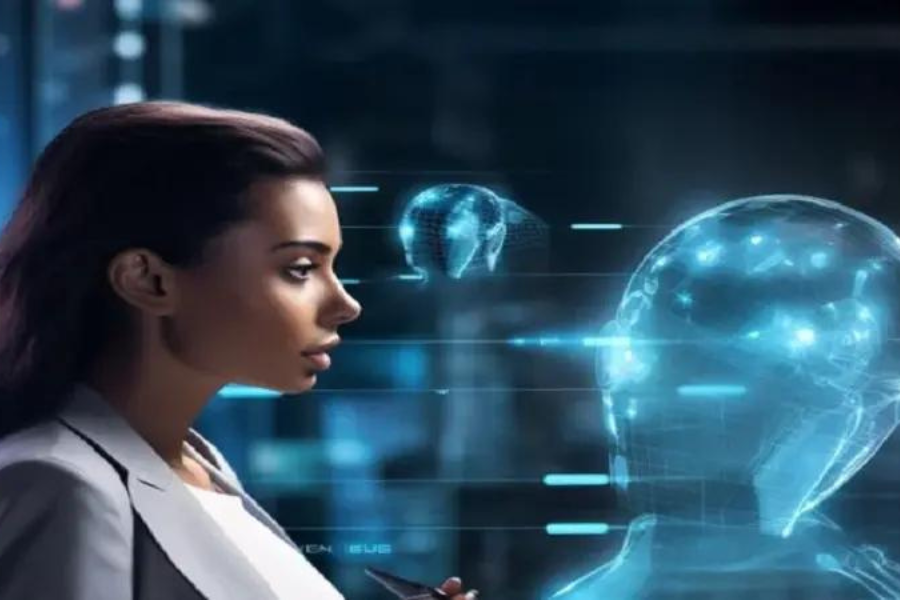
Artificial Intelligence is a field rich with specialized terminology, often conveyed through acronyms that encapsulate complex concepts. Understanding these acronyms is essential for anyone looking to engage with the tech world effectively.
AI (Artificial Intelligence)
At the core of AI terminology is the acronym AI itself. Artificial Intelligence refers to the broad field of technology aimed at creating machines capable of performing tasks that typically require human intelligence. This includes everything from basic rule-based systems to advanced algorithms capable of complex problem-solving.
ML (Machine Learning)
Machine Learning, abbreviated as ML, is a subset of AI focused on developing algorithms that allow computers to learn from and make decisions based on data. Unlike traditional AI systems that follow explicit instructions, ML models improve their performance over time as they are exposed to more data. This learning process enables them to recognize patterns and make predictions or decisions without human intervention.
DL (Deep Learning)
Deep Learning, or DL, represents an advanced branch of Machine Learning. It utilizes neural networks with many layers to analyze large datasets and recognize intricate patterns. Deep Learning is particularly effective in handling unstructured data such as images, audio, and text. Its capability to process vast amounts of data makes it ideal for applications requiring high accuracy, such as image recognition and natural language understanding.
NLP (Natural Language Processing)
Natural Language Processing, abbreviated as NLP, is a critical area of AI that enables machines to understand and interact using human language. NLP powers applications such as chatbots, translation services, and voice assistants by allowing computers to process and interpret natural language in a way that is meaningful and contextually appropriate.
These acronyms form the foundation of AI technology, but there are many more terms and abbreviations that contribute to the rich vocabulary of the field. AlAikas provides a comprehensive guide to help users navigate this complex terminology.
Understanding the Differences Between AI, ML, and DL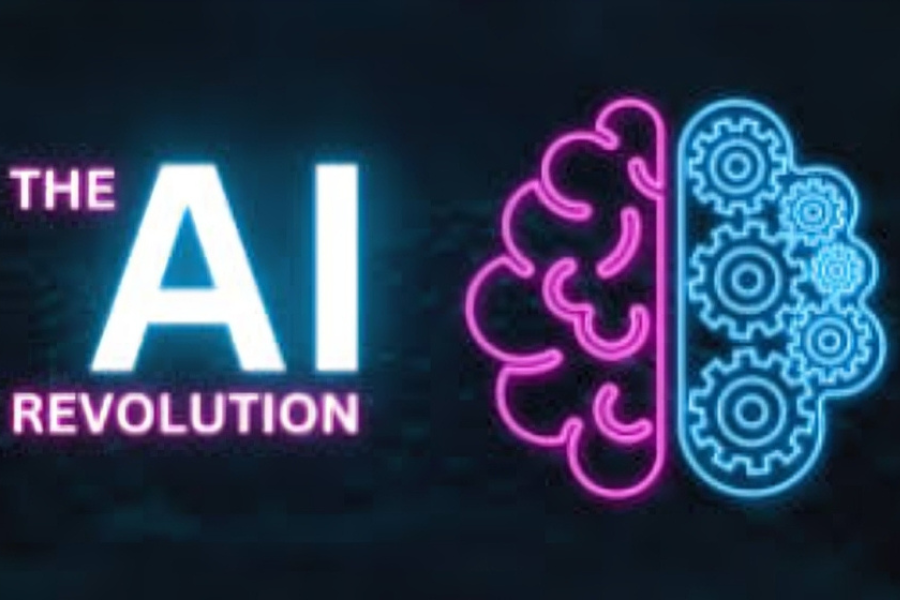
Artificial Intelligence, Machine Learning, and Deep Learning are terms often used interchangeably, but they represent distinct concepts with unique characteristics and applications.
AI (Artificial Intelligence)
AI is the overarching field that encompasses various techniques and methods designed to create machines that exhibit human-like intelligence. It includes both traditional approaches, such as rule-based systems, and more modern techniques like ML and DL. AI’s goal is to enable machines to perform tasks that typically require human cognitive abilities, such as problem-solving, reasoning, and learning.
ML (Machine Learning)
Machine Learning is a subset of AI focused on the development of algorithms that allow computers to learn from data. Unlike traditional AI systems that rely on predefined rules, ML models adapt and improve their performance based on the data they are exposed to. This ability to learn and generalize from examples makes ML particularly powerful for tasks such as pattern recognition and predictive analytics.
DL (Deep Learning)
Deep Learning is a specialized area within Machine Learning that employs neural networks with multiple layers to process and analyze complex datasets. The depth of these neural networks allows DL models to identify intricate patterns and make highly accurate predictions. Deep Learning has been instrumental in advancing fields such as computer vision, natural language processing, and autonomous systems.
Understanding the distinctions between AI, ML, and DL is crucial for grasping how each technology contributes to the broader landscape of artificial intelligence.
The Benefits of Using AI in Various Industries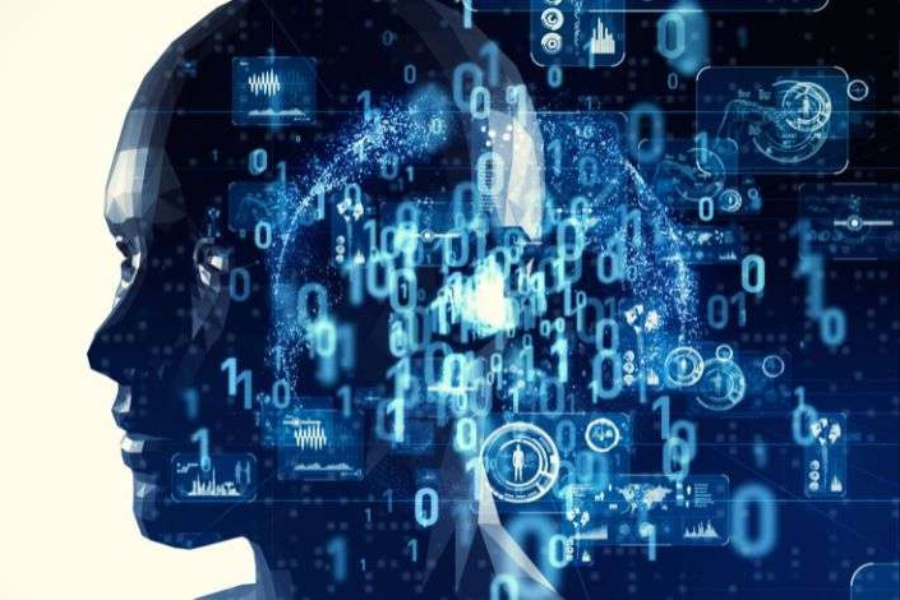
AI has made significant inroads into various industries, offering numerous benefits that enhance efficiency, productivity, and innovation. Here are some examples of how AI is transforming different sectors:
Healthcare
In healthcare, AI is revolutionizing diagnostics and treatment planning. Algorithms powered by AI can analyze medical images with high precision, identifying early signs of diseases such as cancer. This early detection enables timely interventions and personalized treatment plans, improving patient outcomes and reducing healthcare costs.
Finance
The financial sector has embraced AI to enhance fraud detection and optimize investment strategies. Machine Learning models analyze vast amounts of transaction data to identify unusual patterns that may indicate fraudulent activities. AI-powered tools also assist in portfolio management by analyzing market trends and providing insights for better investment decisions.
Retail
AI is reshaping the retail industry by personalizing shopping experiences for consumers. By analyzing consumer behavior and preferences, AI algorithms can recommend products tailored to individual tastes, enhancing customer satisfaction and driving sales. Retailers use AI to optimize inventory management and streamline supply chain operations as well.
Manufacturing
In manufacturing, AI-driven predictive maintenance systems help prevent equipment failures by analyzing data from machinery. These systems can predict when a machine is likely to malfunction, allowing companies to perform maintenance proactively and avoid costly downtime. This approach not only improves operational efficiency but also extends the lifespan of equipment.
Education
AI is making a significant impact on education by enabling personalized learning experiences. AI-powered educational platforms can adapt content to individual student needs, providing customized learning paths and resources. This personalization enhances student engagement and helps educators address diverse learning styles and challenges.
These examples highlight the transformative power of AI across various industries, demonstrating its potential to drive innovation and improve outcomes.
Applications of AI Acronyms in Real-world Scenarios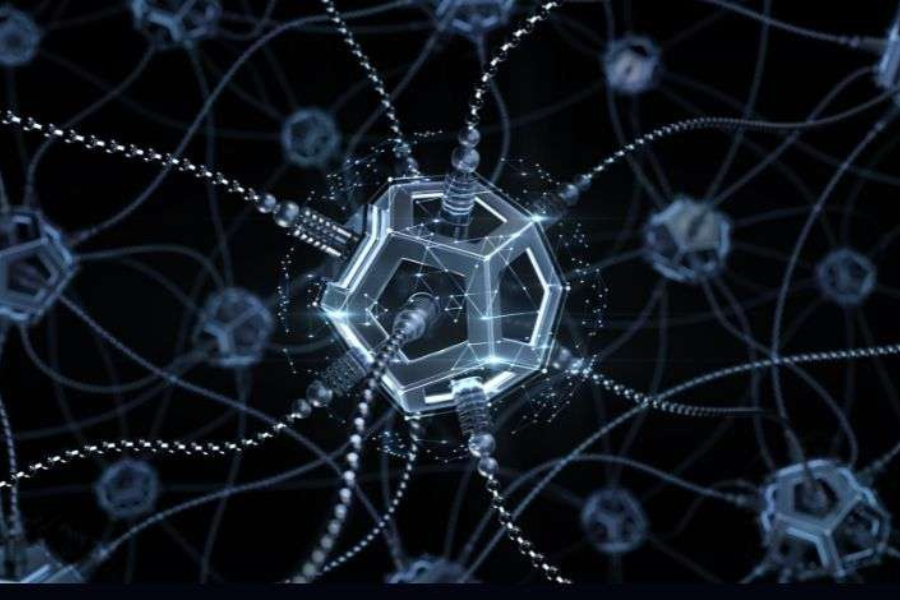
AI acronyms are not just theoretical concepts; they have practical applications that significantly impact our daily lives. Here’s how some of these acronyms are utilized in real-world scenarios:
NLP (Natural Language Processing)
NLP is at the heart of many modern applications. Virtual assistants like Siri and Alexa rely on NLP to understand and respond to user queries. In customer service, AI chatbots powered by NLP provide real-time support, handling routine inquiries and freeing up human agents for more complex tasks. NLP also enhances machine translation, enabling seamless communication across different languages.
ML (Machine Learning)
Machine Learning is widely used in various domains, including finance, healthcare, and e-commerce. In finance, ML algorithms analyze transaction data to detect fraud and assess credit risk. In healthcare, ML models assist in diagnosing diseases and predicting patient outcomes. E-commerce platforms leverage ML for personalized product recommendations and targeted advertising.
DL (Deep Learning)
Deep Learning is transforming fields such as computer vision and natural language processing. For instance, DL models are used in facial recognition systems to identify individuals accurately. In healthcare, DL algorithms analyze medical images to detect abnormalities. Self-driving cars rely on DL for object detection and navigation, enhancing road safety and driving efficiency.
RPA (Robotic Process Automation)
Robotic Process Automation (RPA) is utilized to automate repetitive and rule-based tasks. In administrative settings, RPA can handle data entry, processing, and reporting, reducing the need for manual intervention. This automation improves efficiency and accuracy while allowing human workers to focus on more strategic activities.
These real-world applications demonstrate the practical value of AI acronyms, showcasing how they contribute to advancements in technology and improve various aspects of our lives.
Challenges and Ethical Concerns Surrounding AI Technologies
As AI technologies advance, they bring with them significant challenges and ethical concerns that need to be addressed to ensure responsible and equitable use.
Data Privacy
Data privacy is a major concern in the age of AI. Many AI systems rely on vast amounts of personal data to function effectively. This raises questions about how data is collected, stored, and used. Ensuring that individuals’ data is protected and used ethically is crucial for maintaining trust and safeguarding privacy.
Bias in AI Systems
Bias in AI systems is another critical issue. Algorithms trained on biased or incomplete datasets can perpetuate existing stereotypes and discrimination. This bias can lead to unfair outcomes in areas such as hiring, lending, and law enforcement. Addressing bias requires careful design and testing of AI systems to ensure they are fair and inclusive.
Accountability
Determining accountability for errors made by autonomous systems remains a challenge. When an AI system makes a mistake, it is often unclear who is responsible—the developer, the user, or the AI itself. Establishing clear guidelines and frameworks for accountability is essential for managing the risks associated with AI technologies.
Transparency
Transparency is crucial for building trust in AI systems. Many complex models operate as “black boxes,” making it difficult to understand how they reach their decisions. Increasing transparency involves developing methods to explain AI decision-making processes and ensuring that users can comprehend and challenge the outcomes.
Addressing these challenges is essential for fostering responsible innovation and ensuring that AI technologies benefit society as a whole.
Conclusion: Embracing the Future with AI Acronyms by AlAikas
Artificial intelligence acronyms by AlAikas provide a valuable framework for understanding the complex world of AI. By breaking down these acronyms and exploring their applications, benefits, and challenges, we gain insight into how AI is shaping our future.
Embracing advancements in AI, such as Machine Learning (ML) and Deep Learning (DL), opens up opportunities for innovation across various industries. As we navigate this evolving landscape, addressing ethical concerns and ensuring responsible use will be key to maximizing the positive impact of AI on society.
For those seeking to stay informed and engaged with the latest developments in AI, AlAikas offers a comprehensive guide to understanding and utilizing these acronyms effectively. By demystifying AI terminology, we pave the way for a more informed and empowered interaction with the technologies that are driving the future of our world.
Stay in the know with the latest news and updates on Cnbcblog.com